The growing popularity of large language models (LLMs) has raised concerns about their accuracy. These chatbots can be used to provide information, but it may be tainted by errors or made-up or false information (hallucinations) caused by problematic data sets or incorrect assumptions made by the model. The questionable results produced by chatbots has led to growing disquiet among users, developers and policy makers. The author argues that policy makers need to develop a systemic approach to address these concerns. The current piecemeal approach does not reflect the complexity of LLMs or the magnitude of the data upon which they are based, therefore, the author recommends incentivizing greater transparency and accountability around data-set development.
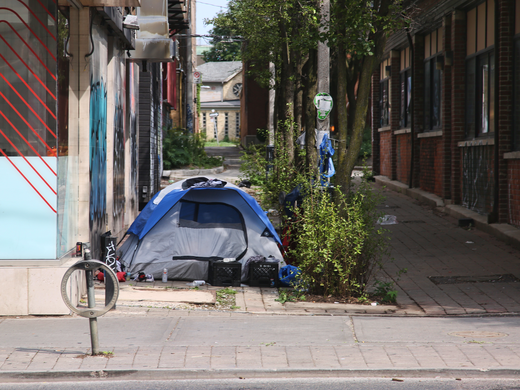