Key Points
- The digital transformation of society is unfolding at a pace that outstrips the development of experience-based policy, raising serious socio-economic risks and commensurately significant socio-economic management challenges.
- At the foundation of these challenges is a pervasive market-failure-inducing information asymmetry, which crosses many divides, including human versus machine, across businesses and between nations.
- The different approaches toward regulation and commitments in international agreements by the major economies reflect self-interest more than systemic considerations. These do not necessarily reflect the needs of the small open economy (i.e., Canada).
- The data-driven economy is fundamentally different than what has come before. A renovation of our economic accounts and the formal economic models used to inform economic policy is needed, in concert with experimentation regarding the design of regulatory frameworks.
he digital transformation is creating a new kind of economy based on the “datafication” of virtually any aspect of human social, political and economic activity as a result of the information generated by the myriad daily routines of digitally connected individuals and machines. The economics of this emerging data-driven economy can be situated in theoretical models of endogenous growth, which introduce research and development (R&D) (Romer 1990), human capital formation (Lucas 1988) and Schumpeterian creative destruction through business stealing (Aghion and Howitt 1992) as drivers of economic growth, together with positive externalities related to local knowledge spillovers. This theoretical framework allows for differential rates of growth in different countries based on their policies to support innovation, such as subsidies for R&D and education to exploit knowledge externalities, but also openness to trade to access technological developments generated elsewhere. It also allows for innovation to generate market power and monopoly rents because, even though knowledge is non-rivalrous (i.e., it can be used simultaneously by many agents without detracting from its utility), it is at least partially excludable (i.e., innovating firms can restrict access to the novel features of their inventions).
However, the data-driven economy has several structural features that make it at least a special case of the general endogenous growth model, if not a new model altogether:
- pervasive information asymmetry;
- the industrialization of learning through artificial intelligence (AI);
- winner-take-most economics, which results in the proliferation of “superstar” firms;
- new forms of trade and exchange, the value of which is not captured by traditional economic accounting systems; and
- systemic risks due to vulnerabilities in the information infrastructure.
Asymmetry as the Foundation of the Data-driven Economy
A fundamental point of differentiation of the data-driven economic model from the knowledge-based economy model from which it emerged lies in the assumption that knowledge is implicitly accessible by all, even if it is temporarily excludable by innovating firms. This does not appear to be true of the information extracted from “big data.”1 To the human mind, big data is meaningless noise; to computers, it is an information mine. It is precisely the ability of computers to extract systematic information out of this noise that underpins the value proposition of big data and the algorithms built on it. Accordingly, information asymmetry between human and machine is at the foundation of the data-driven economy and makes it prone to market failure. Given the significant capital investments required to exploit big data, information asymmetry also applies across firms. Given the digital divide, it applies across countries as well. Information asymmetry and the market failure to which it tends to give rise are fundamental to the sources of economic gains opened by the data-driven economy — they constitute, in this sense, its original sin.
The Industrialization of Learning
A second fundamental point of differentiation of the data-driven economy is the industrialization of learning through the ever-wider deployment of AI (Ciuriak 2018). In the first instance, this promises to accelerate the pace of change and to telescope transformative structural adjustments into a substantially shorter time frame than was experienced previously. This will require rapid institutional responses in areas ranging from labour market adjustment (for example, to address concerns raised by the “gig” economy) to investment planning (for example, to account for shortening of product life cycles, more rapid depreciation of capital investments and a rise in the “hurdle rate” for investment due to greater uncertainty about future earnings). Rapid change points to an increase in the real option value of waiting for more information (Dixit and Pindyck 1994), which implies a paradoxical slowdown in investment at a point of accelerated innovation.
To the human mind, big data is meaningless noise; to computers, it is an information mine.
A second and seemingly larger implication of the industrialization of learning is the discounting of the value of human capital. The futurist Ray Kurzweil has predicted that AI will pass a valid Turing test by 2029 (Galeon and Reedy 2017), thereby marking the point where machine intelligence matches human intelligence.2 The economic significance of this must be considered in light of the accumulated stock of what might be termed “machine knowledge capital,” which is both a complement to and substitute for human capital. In the decade to 2029, this stock will become almost arbitrarily large because the marginal cost of creating the equivalent of a new machine Ph.D.-equivalent will be effectively zero once the first one has been minted.
The implications for the aggregate wage bill going to human capital are troubling. For many jobs that combine several non-specialized tasks, the advent of AI may change how the jobs are carried out rather than eliminating them. For example, while AI might automate long-haul driving, more complex tasks in navigating the short-haul, last-mile segment and performing other tasks such as en route repairs, might still require the services of a human truck driver. Alternative scenarios suggest it is possible that automation of the long-haul segment could actually lead to a more-than-offsetting increase in jobs at the short-haul segment (Madrigal 2018). However, human capital is characteristically highly specialized and thus seemingly more vulnerable to competition from machine knowledge capital (i.e., the substitution elasticity might be substantially higher than for the package of general purpose but low-end skills of a truck driver, resulting in a decline in the wage bill for highly skilled work; see DeCanio 2016). This has very significant implications for the education and innovation policies of advanced countries, whose wealth derives largely from specialized human capital.
Market Concentration, Superstar Firms and Strategic Behaviour
In terms of market structure and behaviour, the data-driven economy, like the knowledge-based economy that spawned it, features economies of scale and network externalities, which give rise to concentrated market structures, expanded economic rents and incentives for strategic behaviour, including in trade policy (as explained by Brander and Spencer 1985). If the technological environment allows the marginal cost of serving additional customers to fall to very low levels, the skewing of market share and rent capture by the suppliers with a quality advantage can be extreme. This is the “winner-take-most” feature of the economics of superstars first developed by Sherwin Rosen (1981).
While these features were perceptible in the knowledge-based economy, they appear to be strongly accented in the data-driven economy due to the characteristics of data. For example, the initial investment cost to capture, assemble and process data is high, but the marginal cost of expanding data assets is very low. Indeed, much of the data now being collected is the by-product of activity using digital infrastructure (“data exhaust”) (Manyik et al. 2011, 1) and the cost of expanding data capital is essentially the cost of expanding storage capacity. As well, the cost of distributing digitized products that help generate the data exhaust is also low, given zero or near-zero marginal production costs for digital products (Rifkin 2014), and near-frictionless commerce enabled by the internet and globalization, which facilitates the more efficient firms to capture greater market share (Van Reenan and Patterson 2017). This makes the economies of scale in the data-driven economy steep. Similarly, the network externalities in the digital realm appear to be powerful, which tends to enable the emergence of natural monopolies or near monopolies, as in the case of search engines (Autor et al. 2017). The intensive use of intellectual property to protect established positions in the data-driven economy creates stumbling blocks for potential challenges (Wagner 2015).
In the United States, concentration increased significantly across a wide swathe of industries: between 1997 and 2012, the weighted-average share of the top four firms’ revenues in each industry, across 893 industries, rose from 26 percent to 32 percent of the total (The Economist 2016). John Van Reenen and Christina Patterson (2017) provide evidence that much of the increase came from the shift of the mass of the economy between firms toward superstar companies. The data-driven economy promises to hasten and intensify this consolidation of market share at the top end of the distribution.
The implications of the winner-take-most feature is set in stark relief by the difference between the market capitalization of two one-time rivals: Google’s market cap closed 2017 above US$700 billion, whereas Yahoo’s was only US$4.5 billion at the time of its final disposition.3 This differentiation in outcomes drives strategic behaviour. As Steven Davidoff Solomon (2016) observes: “Facebook and its elite brethren will do anything to make sure they are not the next Yahoo or Radio Shack, killed by disruption and failure to innovate. This translates into paying obscene sums for technology that might challenge their dominance one day.” While the acquisition of rivals to pre-empt competition has long been part of corporate rivalry, the stakes appear to be much higher in the data-driven economy than previously. In turn, this creates new factual contexts for the administration of competition policy in the domestic sphere and foreign direct investment policy in the international domain.
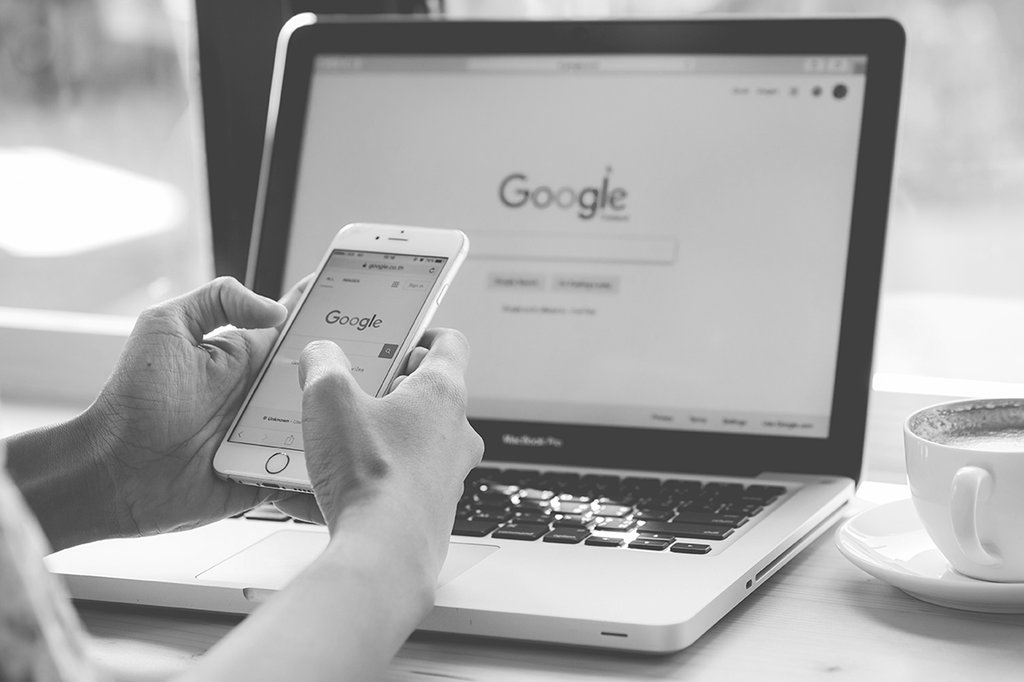
New Forms of Trade and Exchange Value
The uses to which data is put and the roles it plays in society and the economy are as varied as its sources and the entities compiling it. In the data-driven economy, data sometimes is the product itself — as in the case of digitized services — and sometimes it is “exhaust,” the by-product of digital interactions. Sometimes it is monetized and hence its value is recorded in the conventional economic accounts, but in most current uses it is captured without payments and without generating an ensuing paper trail of invoices and receipts. By the same token, its value is significantly understated in existing economic accounting systems (Lawless 2017). It is traded across borders, but in a new mode of barter transaction in which the value on one side is “free” services and on the other is an increment to intangible capital; this escapes capture in trade statistics (Ciuriak and Ptashkina 2018).
It is an open question as to what will prove to be the most robust, secure and efficient architecture for the information society infrastructure in the data-driven-economy era.
Taking implicit exchange values as a guide, one indicator of the value of data is the value of free services acquired by consumers from the internet. Leonard Nakamura, Jon Samuels and Rachel Soloveichik (2017) put this figure at about 1.8 percent of US GDP or in the order of US$300 billion. Looking at the other side of the transaction, this data generates intangible assets for data-driven firms such as Google (year-end 2017 market cap of US$727 billion), Facebook (US$516 billion) and Uber (US$50 billion or so).4 This puts the likely market value of data in its emerging role as the essential capital of the data-driven economy in the trillions of dollars at the dawn of the data-driven-economy era, with potential for even greater expansion as the digital transformation races forward (Ciuriak 2017).
The data-driven economy thus requires renovation of our economic accounts and the formal economic models used to inform economic policy to capture the impact of datafication on measures of economic output and of the factors of production.
Systemic Risk
The data-driven economy is unfolding at a pace that outstrips the development of experience-based policy and experimentation with alternative regulatory models to address systemic risk, including regarding personal data privacy, political manipulation and cyber security. Polar opposite models that are in play are the e-Estonia model with its tight controls on use and storage of personal data and provisions for systemic back-up facilities to guard against hacking (Heller 2017) and the cloud model promoted by the US internet giants in the Trans-Pacific Partnership negotiations, which demands the free flow of data across borders and proscribes data localization.
It is an open question as to what will prove to be the most robust, secure and efficient architecture for the information society infrastructure in the data-driven-economy era. Indeed, the very lack of experience with alternative models and regulatory approaches has led to arguments against the regulation of the digital economy precisely because we do not yet know enough to regulate effectively (Stone et al. 2016). The same rationale applies to treaties that constrain the regulation of the digital economy (Ciuriak 2018).
The major economies are aligning policies in international agreements with perceived national interests: the United States is promoting an open architecture that aligns with the market dominance of its data-intensive firms, whose approach to systemic risks reflects private considerations only; the European Union is promoting sound regulation, which aligns with its primarily defensive interests; and China is taking advantage of the size of its internal market to develop a competitive digital economy. For the small, open economies, the question is whether any of these models are in their interests. Given this, flexibility to regulate in the national interest, without incurring penalties that would tend to generate inaction due to “regulatory chill” effects, seems to be a paramount consideration when making commitments in such agreements (ibid.).
Conclusions
The data-driven economy creates new and significant economic management challenges on many grounds:
- The many layers of asymmetry that are fundamental to the data-driven economy, including between human and machine intelligence, across firms due to the propensity for the dominance by superstar firms and between nations given the digital divide, call into question a laissez-faire approach to national regulation and economic strategies for prospering in the digital age.
- The emergence of machine knowledge capital as a rival to specialized human capital creates secular risk to the asset values that underpin the wealth of economies that have built their niche in the global economy on significant investments in human capital. This rivalry opens up the possibility of significant and politically charged shifts in the balance of returns captured by capital versus labour as machine knowledge capital expands massively at minimal marginal cost in competition with human knowledge capital.
- The tendency for concentration in market structures and attendant strategic behaviour of firms create new factual contexts for competition policy in the domestic sphere and foreign direct investment policy in the international domain.
- Given the new forms of capital that underpin the data-driven economy and new forms of exchange (including an implicit barter trade of free digital services in exchange for data with apparently very high capital asset value), there is a need for new approaches to quantitative economic analysis, including of the value proposition of offers and requests in international trade negotiations over access to data and the terms of procurement contracts that generate valuable data.
- Given the potential for systemic risk in the information society infrastructure that underpins the data-driven economy, experimentation is called for regarding system design. This is a time for regulatory sandboxes, not binding international agreements on data regulation.