The goal of this paper is to present an intuitive summary of computational learning theory, and its application for analyzing the most popular learning algorithms in machine learning, neural networks. This paper assumes no mathematical background or knowledge of machine learning from the reader. Throughout the paper, the important sections are presented using a pyramid approach (that is, in three levels). After introducing each major idea/topic, its policy implications and connection to Canada’s law are discussed. Finally, a summary of Canada’s relevant artificial intelligence law, with a case example, is given.
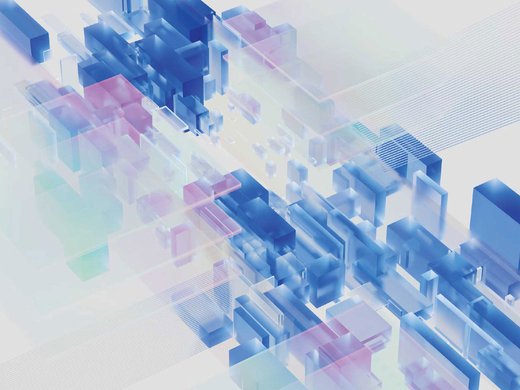